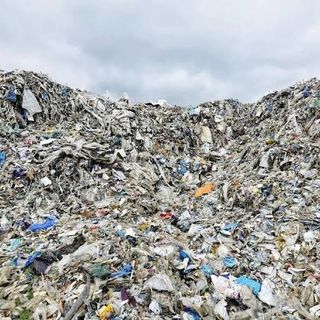
Humans Are Terrible Guessers, and We’re Dragging AI’s Guessing Game Down With Us
However, certain algorithms designed to remove bias-inducing variables from datasets may help coders train machines better.
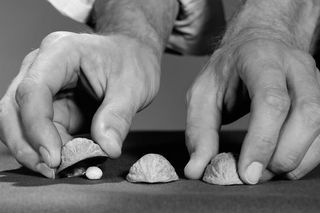
The art of guessing, or finding quick conclusions from available data, has never been a human being’s strong suit. When two paths are presented to us, there is truly no guarantee that we would weigh the pros and cons of each path — almost like a machine — and choose the most accurate one.
Our estimation abilities remain terrible because we are biased creatures. Our inclinations and prejudices are both vital to informing the way we estimate things — but are also the reason why we make inaccurate guesses.
Our biases are often either implicit — i.e., they are what our brains glean from noticing patterns and making generalizations — or they are learned from past experiences or they are influenced by our personality traits, such as optimism and denial. This means that when we do guess, we often forego logical probability and let our biases take control, which means our guesses are often wrong. For example — assuming that individuals of a particular race are more likely to commit crimes because they live in neighborhoods with high crime rates.
Related on The Swaddle:
The Tech Industry’s Sexism, Racism Is Making Artificial Intelligence Less Intelligent
Okay, so if we’re terrible at guessing — are the robots doing any better? Sort of, but not quite. For example — what if we pit humans against algorithms with respect to estimating if people would commit a crime more than once? A new study in Sciences Advances states that algorithms were much better than humans at knowing if an offending individual was likely to get arrested again within two years. Yet, neither the algorithm nor human estimates were close to accurate enough to be trusted completely — which is a dangerous position to be in when it comes todecisions about incarceration.
Algorithms can do a lot better than human beings at assessing large amounts of data very quickly and using it to form accurate guesses. But the problem is, algorithms, and the AI they power, are also rife with biases. Technology is created by human beings, which means it can be both encoded with implicit bias subconsciously and fed biased data, leading to biased solutions. Some examples include how digital assistants on smart devices are often voiced by women (the implicit assumption being that only women are assistants), or how facial identification systems fail at reading an individual’s intent (the implicit assumption being that each emotion only denotes one intent). When it comes to areas like the criminal justice system — already flawed structurally — algorithms built to assess if an individual would re-offend are quite likely to be deeply biased against minority communities. “Rearrest for low-level crime is going to be dictated by where policing is occurring … which itself is intensely concentrated in minority neighborhoods,” Sharad Goel, a computational scientist, told Scientific American.
However, what does help to a certain extent is if algorithms are created to remove variables like that of sex, race, and age in pre-existing data in order to ensure fairness, according to research published in The Annals of Applied Statistics. “What our paper is really doing is a method for taking some data and removing all of the information about race or about sex or about whatever your protected variables are, and then handing that data off to anybody who wants to train an algorithm…The word that people use is that you have ‘repaired’ the data. We have, I think, a more flexible or general way of repairing data. Anything that you produce with it later, it’s not going to have any information about those protected variables anymore,” James Johndrow, who co-authored the research paper, told Knowledge@Wharton.
Failing to nail down a guess accurately enough is human. However, for more serious situations like determining if an individual deserves to leave prison for good, a focus on accuracy and level-headed empathetic judgment over bias is the need of the hour — for both human beings and the way human beings design algorithms. Designing algorithms without bias, and feeding them unbiased, contextual data is what will lead to more accurate estimations.
Aditi Murti is a culture writer at The Swaddle. Previously, she worked as a freelance journalist focused on gender and cities. Find her on social media @aditimurti.
Related
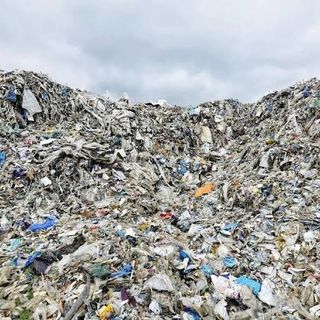
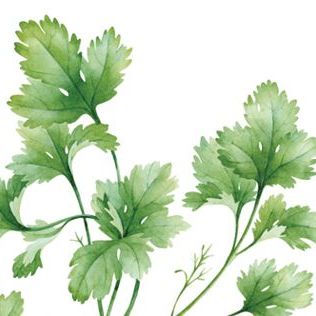
Why Coriander Tastes Like Soap to Some People
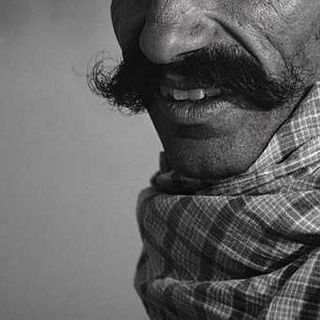